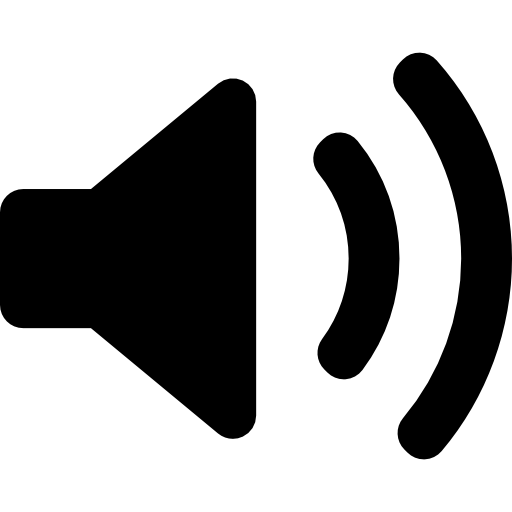
Artificial Intelligence (AI) can reform different areas, from healthcare to finance, from education to entertainment. Notwithstanding, as this extraordinary innovation turns out to be progressively incorporated into our daily lives, it is essential to resolve the issues of inclusivity, fairness, and diversity inside AI frameworks.
Guaranteeing that AI is developed and deployed in a way that regards and advances these principles isn't simply a technological challenge but an ethical objective.
Introduction
Inclusivity in AI implies planning frameworks that are available and valuable to all sections of society, paying little heed to race, orientation, age, handicap, or financial status. Fairness includes guaranteeing that AI frameworks victimize no gathering and that their choices are straightforward and legitimate. Diversity in AI advancement groups and datasets is fundamental to making more adjusted and unprejudiced AI models.
This blog will investigate the importance of inclusivity, fairness, and diversity in AI, analyze the challenges and risks related to one-sided AI frameworks, and examine strategies for creating more equitable AI advances.
Are there ethical considerations?
Artificial Intelligence is when machines can perform tasks that usually require human intelligence. AI systems make decisions or recommendations, which can have a huge impact on people's lives. Without inclusivity, such systems may inadvertently perpetuate existing biases and inequalities. Ethical AI demands that we design our systems with fairness in mind; this means treating each person equally regardless of their background, gender identity, race, and socio-economic status, among other things.
Social Justice
Inclusivity is vital in artificial intelligence for the sake of social justice. AI can reproduce or alleviate social inequalities. For instance, an inclusive hiring process can help identify and correct biases, thus creating equal opportunities for all individuals.
What are the business and economic advantages?
There are also tangible business benefits associated with inclusivity in AI. It is known that different perspectives lead to better problem-solving skills; therefore, diverse teams have been found to be more likely than homogenous ones to innovate around product/service design. Additionally, companies with fairer (inclusive) systems will enjoy a more substantial reputation among customers who will be more satisfied knowing they can access what such company offers regardless of their location or any other form of difference.
What are the challenges in making AI inclusive?
Bias in Data
One challenge is that bias is present in the data used for training AI systems. Societal biases are often reflected in historical datasets; failure to address them can lead to their perpetuation by artificial intelligence. For example, biased training data is the primary reason why facial recognition software has been found to perform poorly on individuals with darker skin tones.
Lack of Diversity in AI Development
Another area for improvement lies in the fact that there has been little diversity within the field of study surrounding artificial intelligence (AI). This means that women, people from minority ethnic groups, and other marginalized communities have been historically underrepresented. Where diversity is lacking, not all users' needs will be fully considered by AIs; this could result in non-inclusive systems. It is, therefore, necessary to ensure varied representation among those involved in designing these technologies if they are to be genuinely inclusive.
Complex and Opaque Algorithms
Many systems using machine learning operate as "black boxes": this signifies that they are difficult to understand because of their complexity and the way decisions are made. In light of this, identifying any biases becomes a challenge, hence making it hard for fairness and inclusivity measures to be put in place.
Strategies towards Fairness and Diversity in AI
Diverse and Inclusive Data Collection
The first step involves getting different types of information together so as not only to ensure that all groups are represented but also to bring out various contexts and settings under which things happen. While doing so, there should be an attempt made at recognizing where biases may have come into play during collection processes then correcting them accordingly.
Bias Detection and Mitigation
Determining and reducing prejudice in AI models is crucial. Some methods can include using algorithms that are sensitive to fairness, regularly auditing systems based on artificial intelligence, and applying methods like reweighting or modifying training data so that they are less biased. Additionally, one should involve diverse stakeholders during evaluation stages since otherwise, some biases may go unnoticed.
Transparency and Explainability
Increasing the transparency and explainability of artificial intelligence systems is essential for inclusivity. When developers make algorithms more interpretable, it becomes easier to understand how decisions were reached and uncover possible biases. This may require utilizing explainable AI (XAI) techniques or developing clear documentation and guidelines regarding these kinds of systems.
Inclusive Design Practices
Adopting inclusive design practices is necessary when creating AI systems that meet everyone's needs. Throughout their design process, creators need to think about accessibility, usability, and cultural awareness.
Such projects not only meet but also exceed people's expectations from different backgrounds; they should involve various user groups while incorporating their feedback into these technologies to ensure inclusivity is achieved at all levels possible.
Promoting Diversity in AI Teams
If you want fair and just artificial intelligence systems, then you must build diverse teams developing them. Organizations should therefore try much as possible to have inclusive hiring processes, offer training programs for minorities in this field while creating an environment where everybody feels like part of the team.
Having different views among colleagues can contribute to more creative solutions because each person brings their own unique perspective based on past experiences, which eventually leads to coming up with unbiased solutions.
Regulatory and Policy Frameworks
It is governments and their associated regulatory orgs that are key to enacting fairness within AI and all its features. The laws and regulations created for this purpose should further bring out honesty, see-thoroughness as well as liability in artificial intelligence systems. For example, such can be done by setting up requirements in relation to how information is collected or even mandatory biased audits, among others.
Case Studies and Examples
Inclusive AI in Healthcare
Within healthcare, an all-encompassing AI approach may be used to counteract differences encountered in medical care and results. For example, the precision of diagnoses for minority groups may be improved by AI models trained with varied datasets.
An illustration is given by an AI system for projecting heart diseases which used data from different demographic categories to ensure it operates with maximum accuracy across all populations.
Fairness in Hiring Algorithms
AI-powered hiring algorithms can help minimize biases during recruitment. However, if not designed inclusively these could perpetuate current prejudice levels. Measures taken by companies such as HireVue ensure that their algorithms are fair when picking employees based on race or gender include utilizing diverse training sets and regularly checking for biases in systems that use them for selecting staff members.
To Sum It Up:
Education and AI become more inclusive through advanced technologies. AI can power personalized learning systems which will take care of individual differences among learners. For instance, Coursera uses artificial intelligence to recommend courses and materials according to one's learning style thus bridging educational disparities.
Conclusion
Not only is it an ethical obligation but also essential for fairness that we make sure our AIs are inclusive. To create unbiased outcomes, we need data without bias, different people developing them with transparency, so everyone knows what they are up against and designs where everyone is involved too otherwise this won't work out.
Everyone from governments down should promote inclusivity in their AI solutions because if we don't do anything about the issue now, then there may never be another opportunity as good or better than today's tech advances towards a fairer world can go unimplemented due to simply lack thereof.
AI mustn't ignore anybody but rather make everybody feel included. It is a voyage that never stops, so through inclusive design, every effort should always be made.
Comments