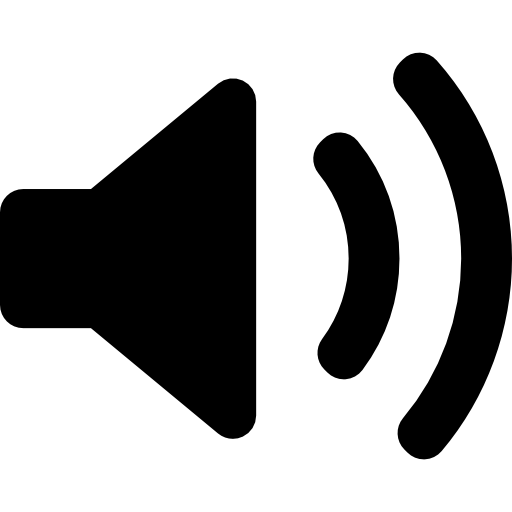
Why is Augmented Analytics Important in Business Intelligence?
In the rapidly evolving world of data, businesses continually seek innovative ways to harness vast amounts of information to drive decision-making and competitive advantage.
The emergence of augmented analytics marks a significant transformation in the business intelligence (BI) landscape. This sophisticated blend of artificial intelligence (AI), machine learning (ML), and natural language processing (NLP) technologies is not only reshaping how data is analyzed but also how insights are gleaned and utilized across various industries.
The Evolution of Business Intelligence
Traditionally, business intelligence has been about collecting data and using various tools to analyze this information. The process often required significant human intervention, from data preparation to analysis. As businesses grew and data became more complex, the limitations of traditional BI tools became apparent. They often could not handle the volume and velocity of data effectively, and insights generation was time-consuming and labor-intensive.
This backdrop set the stage for the rise of augmented analytics. Augmented analytics automates data insights using AI and ML, offering a much more dynamic approach to BI. It is designed to enhance human intelligence, reduce the burden of manual data analysis, and make insights accessible to a broader range of business users, not just data scientists.
Defining Augmented Analytics
Augmented analytics is an approach that uses AI and ML to automate data preparation, insight generation, and explanation. It enables even those without a deep background in data science to understand complex datasets and make informed decisions quickly. This is achieved through advanced algorithms that can detect patterns, trends, and correlations in data that might not be evident to human analysts.
Impact on Decision-Making
One of the primary advantages of augmented analytics is its impact on decision-making. Automating the analysis process provides faster insights, often in real-time. This speed allows businesses to be more agile, responding to changes in market conditions or internal dynamics much more quickly than before.
Moreover, using NLP enables users to interact with data in their natural language, ask questions, and receive answers in a conversational manner, simplifying the decision-making process.
Use Cases Across Industries
The application of augmented analytics is vast and varied across different sectors. Retail, for example, can analyze customer data to predict trends, personalize shopping experiences, and optimize inventory management.
In healthcare, it can process large datasets from patient records to improve diagnostic accuracy and tailor treatment plans. In finance, augmented analytics helps detect fraudulent transactions and manage risk by analyzing historical data and identifying potential red flags.
What are the Challenges and Considerations?
Despite its many benefits, the adoption of augmented analytics comes with its own set of challenges. Data privacy and security are major concerns, as businesses must ensure that the use of AI in data analysis complies with all relevant laws and regulations.
There is also the risk of AI bias, where algorithms might generate skewed insights if the underlying data is biased. Therefore, continuous monitoring and adjustment of AI models are essential to maintain the integrity and accuracy of the insights provided.
What are the challenges and considerations?
Despite its many benefits, the adoption of augmented analytics comes with its own set of challenges. Data privacy & security are major concerns, as businesses must ensure that the use of AI in data analysis complies with all relevant laws and regulations.
There is also the risk of AI bias, where algorithms might generate skewed insights if the underlying data is biased. Therefore, continuous monitoring and adjustment of AI models are essential to maintain the integrity and accuracy of the insights provided.
Enhancing User Engagement Through Augmented Analytics
Augmented analytics streamlines the data analysis process and enhances user engagement. By providing interactive and intuitive analytics tools, businesses can empower employees to participate more actively in data-driven decision-making.
This user-friendly approach encourages a culture of analytics throughout the organization, promoting better understanding and adoption of data insights in daily operations.
What are the prospects of business intelligence?
Looking ahead, the future of BI in business intelligence seems promising. As AI technologies evolve, they will become even more sophisticated, further enhancing their ability to analyze complex datasets and generate actionable insights.
Integrating emerging technologies like blockchain and the Internet of Things (IoT) could also open new avenues for data analysis, making augmented analytics an even more integral part of business intelligence.
Conclusion
The rise of augmented analytics signifies a paradigm shift in how businesses approach data and decision-making.
By leveraging AI, ML, and NLP, augmented analytics not only enhances the efficiency of data analysis but also democratizes access to insights, enabling a more informed decision-making process across all levels of an organization.
As we move forward, the role of augmented analytics in business intelligence is set to grow, fundamentally transforming how businesses operate and thrive in a data-driven world.
This evolution is about technological adoption and how businesses can strategically align these tools to drive real value. In the era of big data, augmented analytics offers a pathway to not just survive but to excel by turning data into one of a company's most valuable assets.
Comments